Challenge: Conduct a 3-month user research study to gain an understanding of how users react to BestReviews's new personalized product recommendation feature. Identify what works and what doesn't based on an analysis of the qualitative data gleaned.
Role and Key Contributions: While I had some guidance from the Product lead and product manager senior to me, the research study was entirely designed and run by me. I designed the study, screened and recruited users, conducted all 17 interviews, analyzed and synthesized findings and presented my recommendations based on these insights.
Outcome: The personalized recommendation feature was a priority product development initiative for 2018. My qualitative study as well as A/B test results would inform the overall impact of this feature and the decision to continue pursuing and developing this idea into 2019. I was not present for the final decision but active deployment of the feature has been observably halted in 2019.
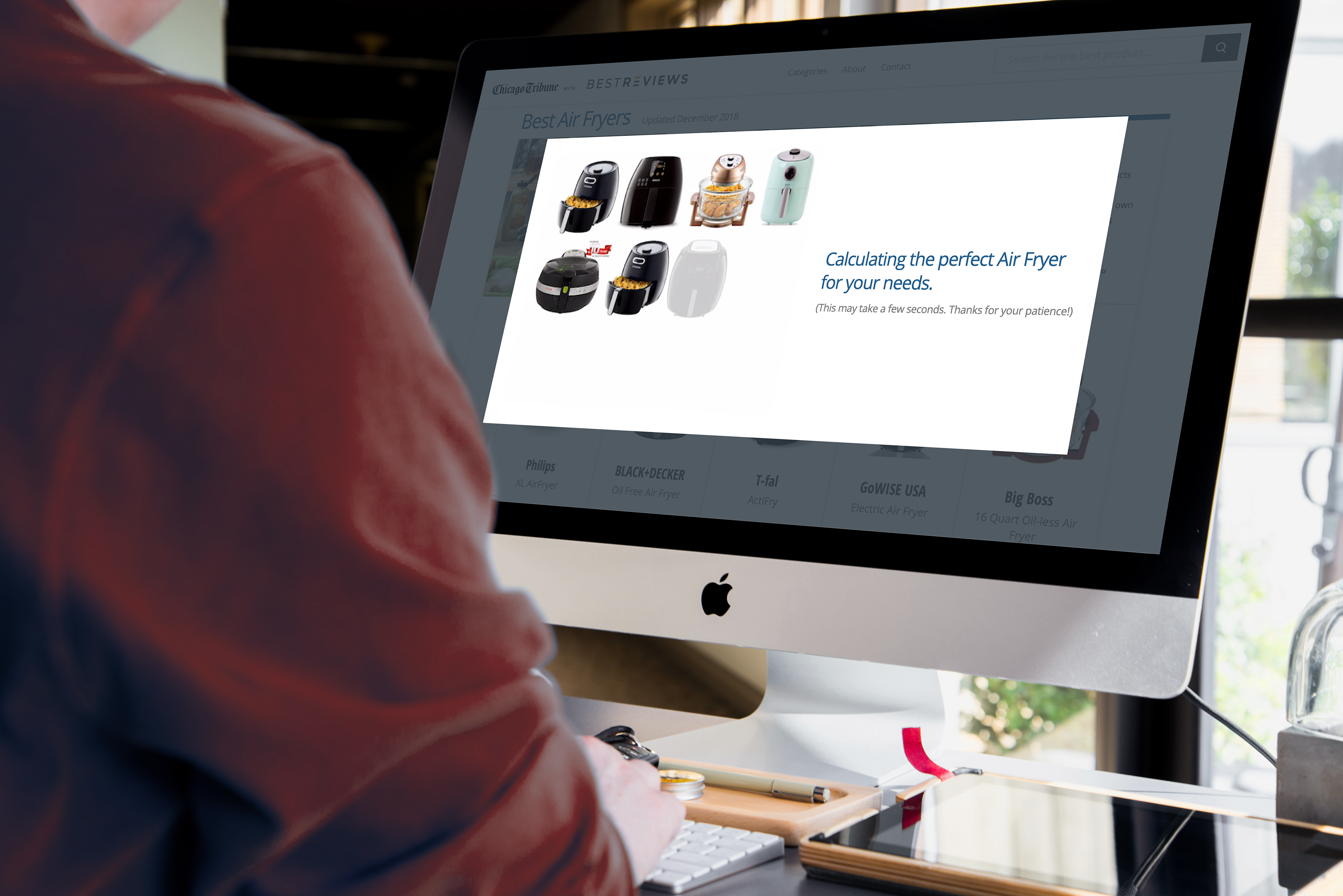
BestReviews is a product review company based in San Francisco, CA. It's business model is built on the affiliate marketing partnerships established by e-commerce retailers. For example, if a customer clicks on a link to a site like Amazon for a product recommended on BestReviews's site and they make a purchase there, BestReviews gets a slice of the profits from Amazon. This model has been widely successful for many content businesses and has since been adopted as a revenue stream for media companies. Notably, Wirecutter was acquired by the New York Times and BestReviews is similarly majority owned by the Tribune Publishing company.
As a product management intern, I was tasked with conducting a quarter-long qualitative study of user reactions to BestReviews's newest feature, a quiz-like personalized recommendation tool. Dubbed internally as the "wizard," it was the primary product development initiative of 2018. BestReviews began a soft roll-out of this feature on the site's "evergreen" pages, or the product pages that had the most consistent purchasing activity. While A/B tests of these features on these pages would offer a quantitive picture of how this new feature was impacting performance and behavior, BestReviews leadership wanted to get a qualitative view of how users were reacting to this feature. Moreover, early A/B testing results was giving a somewhat mixed reaction. A user study would help pinpoint what may be working or otherwise in the experience.
Below are screenshots displaying what the personalized recommendation tool looked like.
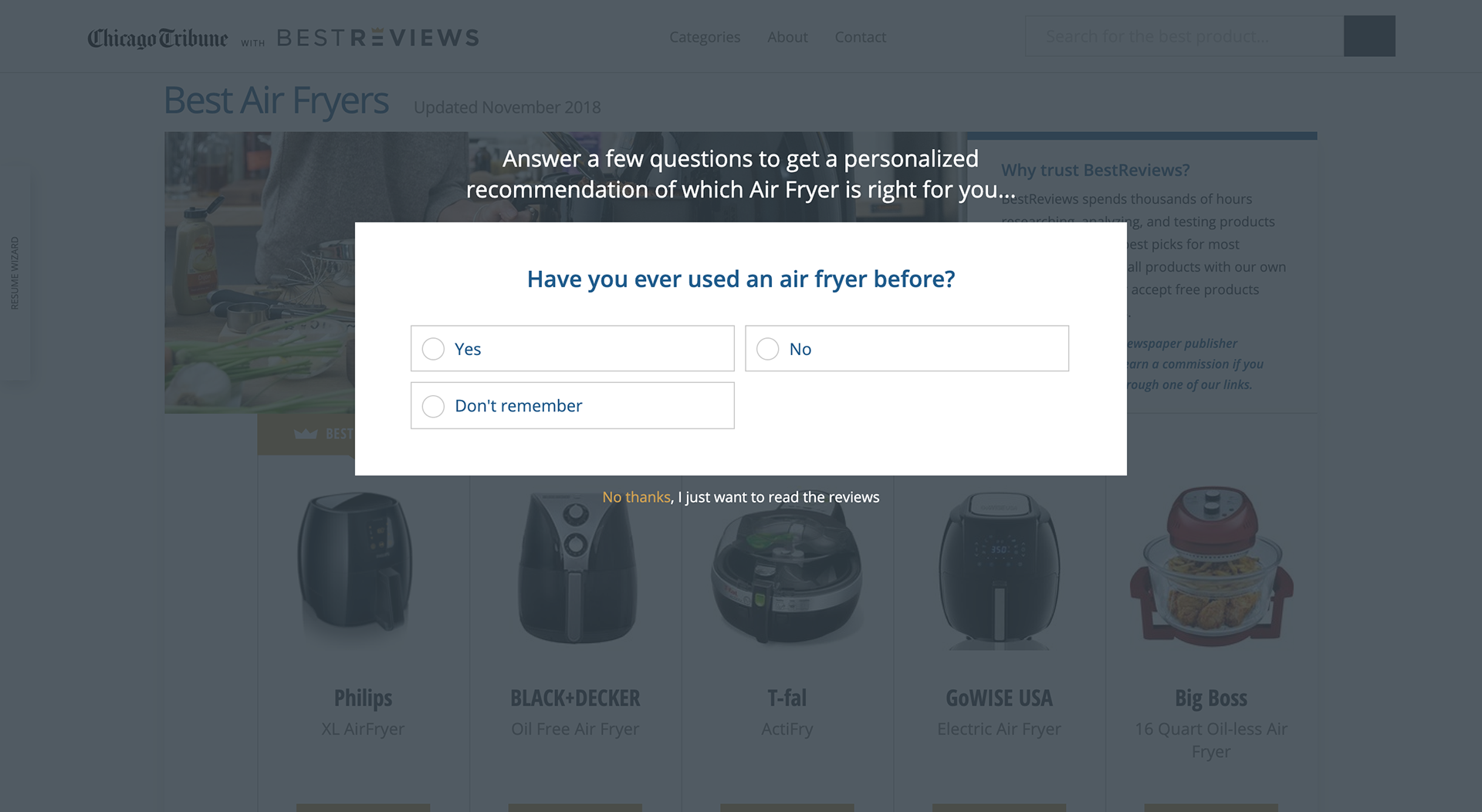
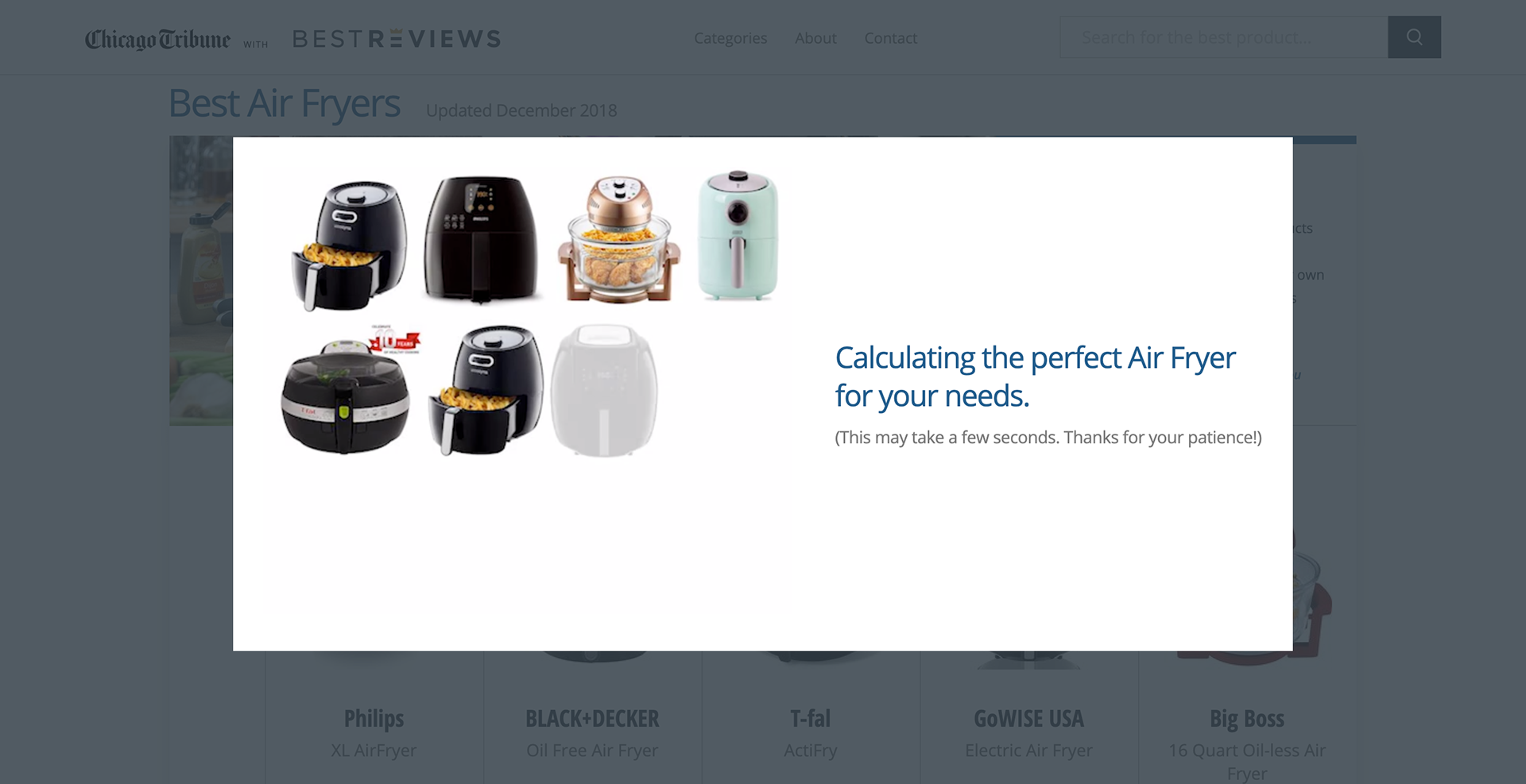
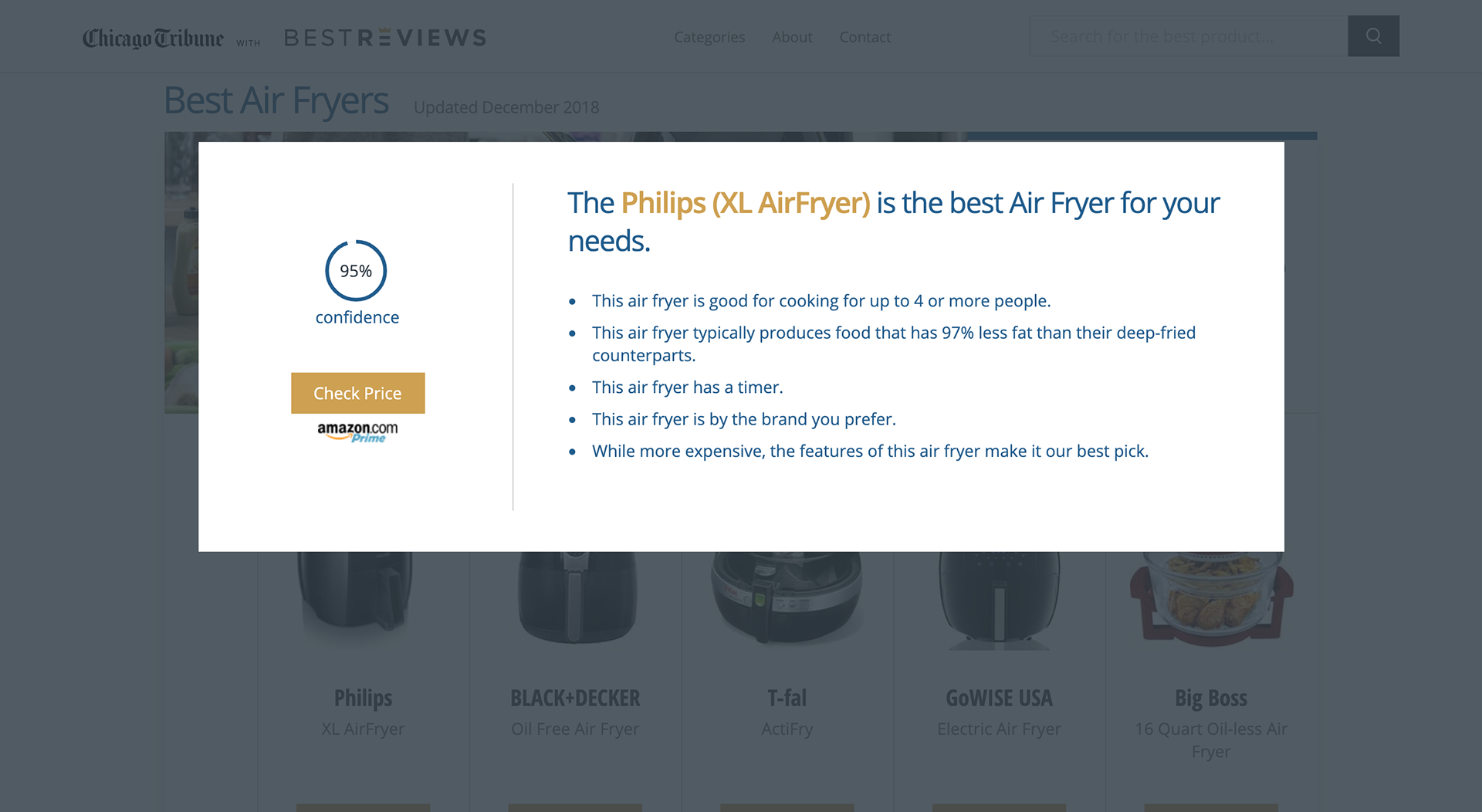
The basic design of the study was to present users with pages from our site that had the feature loaded onto them and to observe their behaviors. Users were not told that the feature was what we were testing for. A limitation in this testing environment is that it could not emulate the real-world conditions of discovering a page based on organic intent. To get as close as possible, I presented a bank of categories users could choose from. I formed three banks, one that had all possible pages, one that had pages that showed positive impact from A/B testing and one that had pages with negative impact according to the A/B tests. As the users explored and interacted with the page, I recorded their behaviors and decisions (using GoToMeeting's screen share and recording function) and asked them to narrate their thoughts.
In terms of recruiting and screening, I launched a recruitment survey through HotJar. Through a simple questionnaire that included demographic information and simple attitudinal/behavioral questions, I screened for a test sample that would be a balance between being reflective of BestReviews's core user-base as well as including relatively extreme cases (outliers).
The following deck is a slightly abridged version of my presentation. It outlines key insights and recommendations for improving the "wizard."